Fantasy Football Team Usage Trends
We looked at how teams have recently distributed touches, yards, and scores among their fantasy-relevant players as part of our projections.
May 12, 2023 - by Jason Lisk
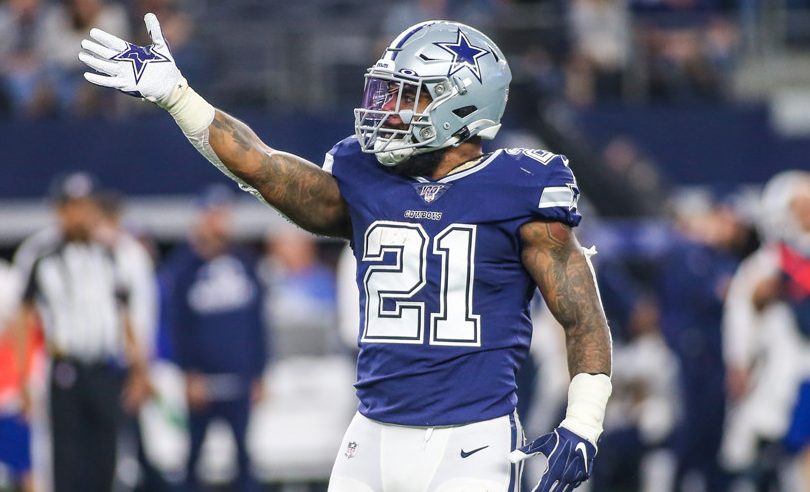
How will Ezekiel Elliott be used by the Cowboys in 2020? (Photo by George Walker/Icon Sportswire)
When creating our fantasy football projections, in addition to player similarity scores we also considered team usage trends.
Team usage trends show how teams have distributed their stats among the fantasy-relevant players on the roster in recent seasons. For example, some teams split their rush attempts fairly evenly between a committee of backs, while others tend to give the starter the bulk of the work. Different teams have different distributions of passes thrown to wide receivers, tight ends, and running backs. While the past is not absolutely predictive of the future, it can be informative.
Creating Fantasy Football Team Usage Trends
We went through all 32 teams and calculated weighted usage trends for the last three years, for offensive roles at running back, wide receiver, and tight end. (For those unfamiliar with fantasy roles, the top running back on a team is called the RB1, and the backup is the RB2. The top wide receivers are the WR1, WR2, WR3, and so on.)
The first step was determining role assignments — which player was the RB1, for example? We wanted to do this in a data-driven, automatic way. However, injuries are part of the game, and teams experience different levels of injury luck from year-to-year. If we just went by raw yard or touch totals, we might incorrectly assign the wrong person to a role. The star wide receiver who played six games might appear to be the WR3, for example, going by totals. But that would not have accurately reflected the team situation.
How We Determined Which Player Would Be Assigned to Which Team Usage Role
Our solution was to look at the highest per-game producers at each position, and assign them to roles, in order. For example, the top wide receiver in targets per game would be the WR1, even if he didn’t play the full 17 games. Of course, our WR1 numbers for that team would be low if we just stopped there. In real life, if a player is out hurt, someone else steps up into that role.
So our WR1 numbers need to consist of 17 games worth of production from the top wide receivers on the roster. If the top wide receiver played every game in a year, the WR1 role would simply be assigned his season stats. But if he missed 8 games, the WR1 role would get his 9 games worth of stats, and then the remaining 8 games would be filled by using 8 games of the next receiver’s stats (prorated from his full season stats). We continued with that same method moving down to WR2, WR3, and so on. The same method was also used at running back and tight end.
The overall usage trends you see below represent our estimate for the percentage of offensive production of the best WR. It represents our estimate of how much the RB1 was used in the running and passing game across the league. But the identify of who that running back or receiver was for a particular team could change from game-to-game based on injuries. The WR1 for a team in a given year could be based on the stats of only one player, or it could be a mix of two or more players.
NFL League-Wide Team Usage Trends
With that said, we thought you might like to see the overall league averages. First here is the data for the 2020 to 2022 seasons.
POSITION | RUSHING ATTEMPTS | RUSHING YARDS | RUSHING TDS | RECEIVING TARGETS | RECEPTIONS | RECEIVING YARDS | RECEIVING TDS |
---|---|---|---|---|---|---|---|
RB1 | 50.5% | 51.3% | 49.0% | 9.2% | 10.7% | 7.3% | 6.0% |
RB2 | 24.3% | 23.2% | 19.7% | 6.3% | 7.2% | 4.7% | 4.0% |
RB3 | 6.4% | 6.0% | 4.9% | 3.6% | 4.0% | 2.8% | 2.6% |
WR1 | 0.9% | 1.2% | 1.6% | 24.1% | 23.4% | 26.6% | 25.1% |
WR2 | 0.7% | 0.9% | 0.7% | 17.0% | 16.1% | 18.8% | 18.2% |
WR3 | 0.8% | 1.1% | 1.2% | 11.7% | 10.9% | 12.4% | 11.9% |
WR4 | 0.7% | 0.9% | 0.6% | 5.8% | 5.2% | 5.8% | 4.9% |
TE1 | 0.1% | 0.1% | 0.5% | 14.8% | 14.9% | 14.7% | 17.7% |
TE2 | 0.1% | 0.1% | 0.3% | 5.4% | 5.7% | 5.3% | 6.7% |
And for comparison, here is the data we originally posted three years ago, for 2017 to 2019.
Position | Rushing Attempts | Rushing Yards | Rushing TDs | Receiving Targets | Receptions | Receiving Yards | Receiving TDs |
---|---|---|---|---|---|---|---|
RB1 | 53.7% | 53.8% | 52.4% | 9.4% | 10.9% | 7.8% | 6.3% |
RB2 | 24.0% | 23.1% | 21.7% | 7.6% | 8.8% | 6.3% | 5.3% |
RB3 | 6.7% | 6.5% | 6.1% | 4.0% | 4.6% | 3.3% | 2.0% |
WR1 | 0.8% | 1.2% | 0.6% | 23.4% | 22.5% | 26.2% | 26.2% |
WR2 | 0.6% | 0.9% | 0.5% | 16.9% | 15.8% | 18.1% | 18.2% |
WR3 | 0.5% | 0.7% | 0.4% | 11.8% | 10.5% | 11.7% | 11.3% |
WR4 | 0.5% | 0.8% | 0.6% | 5.6% | 5.1% | 5.6% | 4.7% |
TE1 | 0.1% | 0.1% | 0.2% | 14.2% | 14.5% | 14.3% | 16.4% |
TE2 | 0.0% | 0.1% | 0.0% | 5.7% | 5.8% | 5.6% | 7.4% |
We can see some interesting recent trends in this data, by comparing how things have changed in just three years. One of the more noticeable is the drop in running back carries, yards, and touchdowns. The primary culprit here is that QBs are now rushing the ball more, and scoring more rushing touchdowns in the most recent three seasons.
Another more subtle one is that teams are more willing to get creative and give carries to wide receivers and tight ends near the goal line. From 2017 to 2019, the receiving positions accounted for 2.3% of all rushing touchdowns. In the most recent three years, that has more than doubled, to 4.9%.
Manually Adjusting Some Team Usage Trends
For most teams, we used their specific three-year weighted average as a guide when making projections. That was often good enough, particularly for teams with the same coach, quarterback style, and similar personnel. But for teams with major changes, looking at recent historic trends may not be as helpful.
Using more years is better from the standpoint of reducing the impact of outlier seasons (particularly when it comes to rushing or receiving touchdown spikes by a certain player). But sometimes more is less. So we made the call on some teams to adopt different approaches, and this was often the case with teams with less history with the same coach and key offensive personnel.
One example for the upcoming 2023 season is Indianapolis, where the team hired Shane Steichen, the offensive coordinator in Philadelphia, and drafted a 21-year-old rushing quarterback in Anthony Richardson, a year after veteran Matt Ryan was the season-opening starter. You can expect that the Colts’ rushing distributions and percentage of carries and touchdowns scored by the QB position will shift dramatically, and potentially look more like what happened in Philadelphia with Steichen and Jalen Hurts than how the Colts have played recently.
Re-Adjusting the Usage Trends to Project Injury Averages
Finally, when we got down to incorporating the team usage trends into our player projections, we had to account for the risk of injury in the future. If you are projecting players to play all 17 games, you are going to be too high in your estimates, on average. For example, the top running backs in recent years have played closer to 14 games as the median result. Some get hurt, some get through the season playing all or most games, and it’s hard to predict who will be in each group.
So we first assigned specific players to team roles, and allocated the percentage of touches, yards, and touchdowns based on those roles. But we then reduced players’ total production down some to account for injury risk.
The Team Usage Trends Reflect the Median of a Range of Injury Outcomes
Take Derrick Henry in Tennessee. The RB1 position for the Titans has a weighted average of 69.6% of rush attempts, 69.8% of rushing yards, and 70.4% of rushing touchdowns. But we are not projecting Henry to be RB1 for all 17 games, due to the risk of injury. Given both the average risk for injury for a RB1, and Henry’s age and potential for decline based on some of his player comps, we project him for closer to 58% of the production overall.
In reality, a whole host of outcomes could occur. They range from Henry playing all 17 games on one end, to Henry getting hurt early in the season at the other. We are trying to project a middle-of-the-road outcome. That’s why we project every player to potentially miss some games. The specific game projection for each was based on the position played and general trends at the position, along with player age and injury history, and games played by comparable players.
So all of our final usage projections do take into account potential missed time for the players. If we did not do that, we would over-project the starters, and have too big a gap in stat distribution among players on a roster.